Invited Speaker
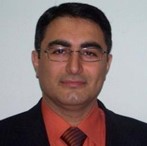
Dr. Khaled Almustafa, Associate Professor
Prince Sultan University, Saudi ArabiaSpeech Title: Prediction of Heart Disease and Classifiers’ Sensitivity Analysis
Abstract: Background: Heart disease (HD) is one of the most common diseases nowadays, and an early diagnosis of such a disease is a crucial task for many health care providers to prevent their patients for such a disease and to save lives. In this paper, a comparative analysis of different classifiers was performed for the classification of the Heart Disease dataset in order to correctly classify and or predict HD cases with minimal attributes. The set contains 76 attributes including the class attribute, for 1025 patients collected from Cleveland, Hungary, Switzerland, and Long Beach, but in this paper, only a subset of 14 attributes are used, and each attribute has a given set value. The algorithms used K- Nearest Neighbor (K-NN), Naive Bayes, Decision tree J48, JRip, SVM, Adaboost, Stochastic Gradient Decent (SGD) and Decision Table (DT) classifiers to show the performance of the selected classifications algorithms to best classify, and or predict, the HD cases. Results: It was shown that using different classification algorithms for the classification of the HD dataset gives very promising results in term of the classification accuracy for the K-NN (K=1), Decision tree J48 and JRip classifiers with accuracy of classification of 99.7073%, 98.0488% and 97.2683% respectively. A feature extraction method was performed using Classifier Subset Evaluator on the HD dataset, and results show enhanced performance in term of the classification accuracy for K-NN (N=1) and Decision Table classifiers to 100% and 93.8537% respectively after using the selected features by only applying a combination of up to 4 attributes instead of 13 attributes for the predication of the HD cases. Conclusion: Different classifiers were used and compared to classify the HD dataset, and we concluded the benefit of having a reliable feature selection method for HD disease prediction with using minimal number of attributes instead of having to consider all available ones.
Keywords: Heart Disease (HD), Prediction, Classification, K-Nearest Neighbor, Support Vector Machine (SVM), Decision tree J48, Feature Selection, Sensitivity Analysis.
Biography: Dr. Khaled Almustafa received his B.E.Sc. in Electrical Engineering, M.E.Sc. and Ph.D. in Wireless Communication from the University of Western Ontario, London, Ontario, Canada in 2003, 2004 and 2007 respectively. He is currently working as an Associate Professor at Prince Sultan University (PSU) in the Department of Information Systems (IS) at the College of Computer Science and Information Systems (CCIS), Riyadh, K.S.A. He served as a General Supervisor for the Information Technology and Computer Services Center (ITCS) at PSU, Chairman of the Department of Communication and Networks Engineering (CME), and the Vice Dean for the College of Engineering at PSU, and the Director of the Research and Initiatives Center (RIC) at PSU. Currently he is the Chief Information and Technology Office (CITO) at PSU.
Research Interests: error performance evaluation of MIMO communication systems in partially known channels, adaptive modulation, and Channel Security, text recognition models, control systems with renewable energy applications as well as Machine and Deep learning, Features Selections and Data Prepossessing.